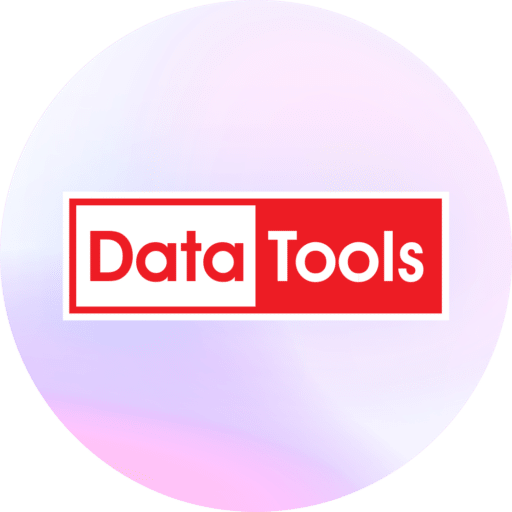
Data Accuracy in Australia & SMS in the Early 2000s
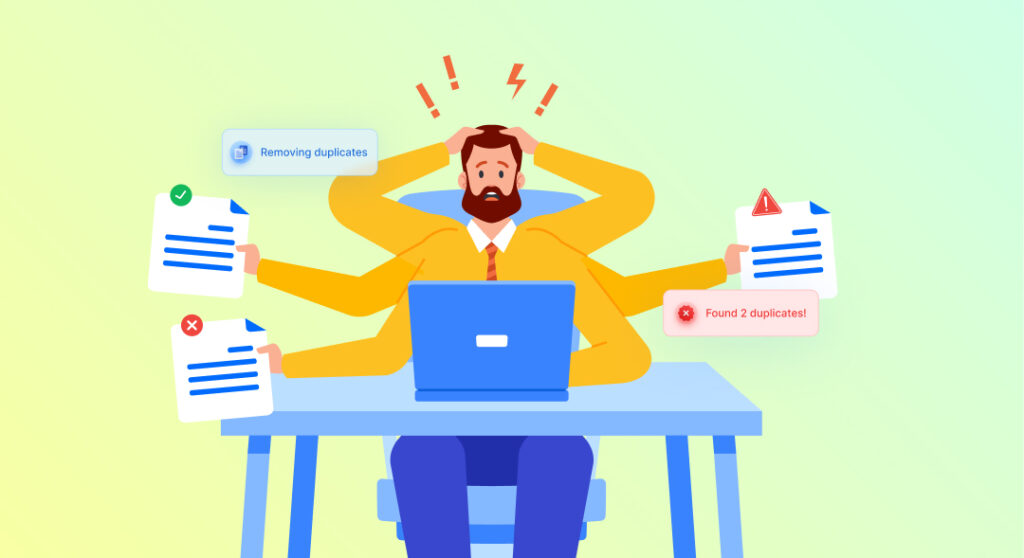
Pretend you’re a teenager in the early 2000’s with one of those keypad phones that could literally stop bullets.
You’re getting ready to meet your textmate of several weeks, and they’ve agreed to meet you at a nearby mall this Saturday.
Look out 4 a tall-ish brunette, their last SMS reads, wearing a yellow blouse n skinny jeans LOL
No problem! On the day, you show up and spend the next forty minutes scanning the crowd closely, growing more and more fidgety by the minute.
Let’s end that on a good note and say that you finally met up with them and struck up a lifelong friendship.
But in 2024, you wouldn’t need to worry about meeting your online buddy with only a vague idea of who they are and what they look like. If you’ve ever tried looking for friends or dates online, you already have a few tricks up your sleeve. You’ve read through their profile, looked them up on social media, and invited them to a video call.
Table of Contents
Fuzzy logic to the rescue
What the teenagers of yore had to go through was the pains of executing a kind of fuzzy logic on their own. This refers to identifying duplicates by looking for nearly matching records, and not just entries that are exact matches.
For anyone who has had to deal with CRMs systems, customer forms, or data entry, this feature is a godsend. Imagine having more than a thousand leads, but more than half of it is a duplicate of another entry written in a slightly different way, misspelled, or formatted wrong.
It would drive anybody nuts!
Working with poor data quality
Previously, the solution to this problem would vary by industry. Telemarketers would just remove duplicate phone numbers. This wouldn’t weed out duplicates from different households, but they would let it pass because it offered more chances to make contact.
People who needed to conduct mail campaigns would combine parts of addresses to help remove duplicates, but the main issue there is it wouldn’t catch vanity suburbs.
When looking for duplicates, businesses need to know the answer to just two main points:
- What fields are available within the data?
- What will the data be used for?
And other points for consideration include:
- Data consistency, or the lack of it
- Data entry errors like keying the street number before the unit number
- Misspelled information.
An answer that’s quick, easy, and reliable
So, what would the ideal solution look like?
Maybe something that could just sit on someone’s desktop and super speedily use fuzzy logic to approximate records that are the same. From there, it would present them to the user so that they can decide whether the fields match, don’t match, or need to be removed from the system entirely.
This is what could happen to improve existing data. But once everything that’s in the database is clean, businesses could also consider verifying data at the point of entry.
This means having a tool in place that watches while customers type in their information in customer forms or subscription forms, and then corrects them as they fill it out. It’s a great way to ensure bad data never enters the system in the first place!
Additionally, something that could craft a unique identifier that would allow people to access and compare data in the future without having them go back to reanalyse the entire dataset would be super helpful.
It’s a smart, time-saving approach, ensuring that if the data stays the same, these keys can be reused, streamlining your processes.
You make the rules for data dedupe
Now, the final consideration is: does the data deduping go over or under?
And no, we’re not referring to a limbo pole!
Navigating automated deduplication tech comes with its trade-offs: choosing between tolerating some duplicates or potentially losing bits of data during cleanup. That’s what we mean: under-cleanse the data to keep everything, including the dirt, or over-cleanse the data and risk losing a few good parts.
We often advise businesses to blend these strategies, adapting to the scenario at hand. For instance, a bit of duplication might be okay for the prospect list, but when it’s time to reach out, it might help to have something that can fine-tune your data.
This would make sure that messages via mail or phone are precise and targeted, to maximise the effectiveness of the campaign.
When cleaning up prospect lists, it’s usually best to over dedupe. This way, the marketing team never has to worry about sending new customer promos to the existing clientele.
That could be a HUGE problem! By using identifiers like phone numbers or addresses, and tossing in a few more data points for accuracy, these lists stay clean and all messages are landing where they should.
It’s time to make data deduping simple
Our sophisticated online and social media interactions are an incredible show of how far we’ve come from SMS adventures and snail mail. And in this age, where data drives decisions, having a clean, organized database isn’t just nice to have—it’s essential.
The right tools not only simplify our lives but also amplify our efforts, ensuring we’re reaching the right people at the right time, without the clutter of duplicates or the risk of overlooking valuable connections.
So, if you’re ready to take the guesswork out of your data management and give your CRM a much-needed facelift, it’s time to consider a solution that’s as forward-thinking as you are.
Get in touch with us today to learn more about our solutions and take the first step towards a cleaner, more efficient database.